Unlocking Production Line Efficiency with
This mid-sized manufacturer reduced machine idle time by 18% and improved on-time orders by 25% through an AI-powered scheduling solution — without replacing their ERP system.
When Growth Meets Scheduling Chaos
A growing Midwest manufacturer of industrial-grade components was facing a common issue: success brought complexity. They ran multiple short-run jobs across several work centers, relying on printed schedules and manual updates to sequence work.
Their ERP generated static job lists, but it couldn’t adapt to rush orders, machine downtime, or real-time floor changes. As a result:
- Static ERP job lists didn’t reflect real-time shop floor realities
- Planners spent hours each day manually sequencing jobs across machines
- Machines were underutilized due to poor handoff timing and changeovers
- Last-minute rush orders disrupted schedules and delayed deliveries
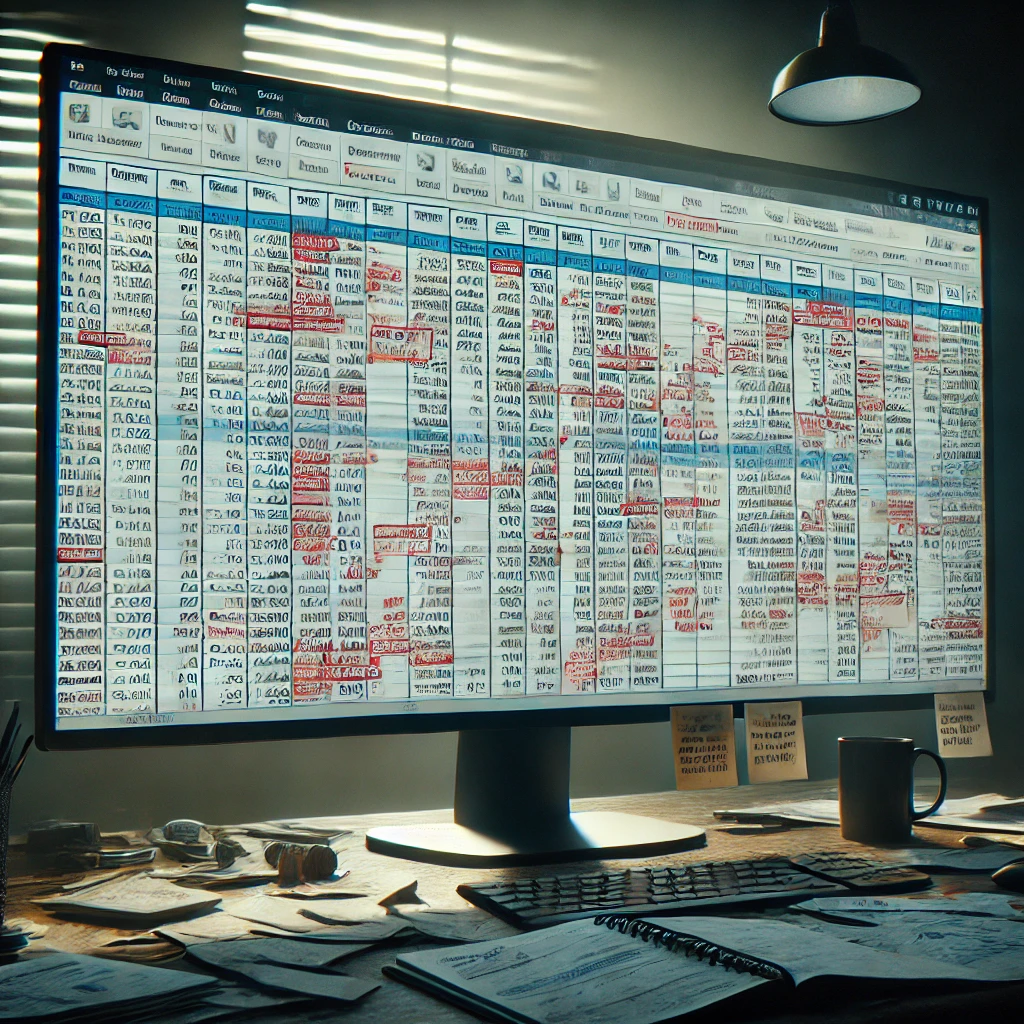
Steps to Success
Captivix first delivered a working AI pilot for intelligent scheduling using the client’s historical data. After validating results with real teams on the shop floor, we scaled the model into a fully integrated scheduling engine — now driving production efficiency every day.
1. Use Case Definition & Pilot Scope
The client approached Captivix with a clear need: improve production scheduling efficiency without overhauling their existing ERP.
Together, we scoped a pilot project focused on:
- Reducing machine idle time
- Improving on-time order completion
- Automating job sequencing with minimal human intervention
- Prioritized BOM optimization as the most impactful and feasible use case
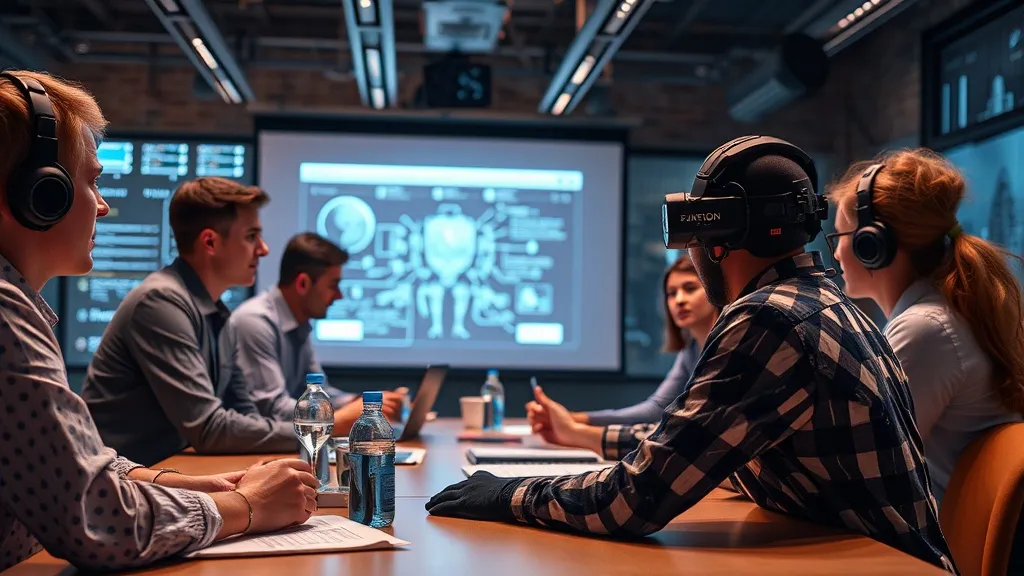
2. Data Review & Feasibility Assessment
To ensure the AI model could learn real-world patterns, our team:
- Collected 3 years of production data, including job histories, shift schedules, and downtime logs
- Analyzed ERP-generated schedules vs. actual shop floor performance
- Cleaned and structured the data for model training
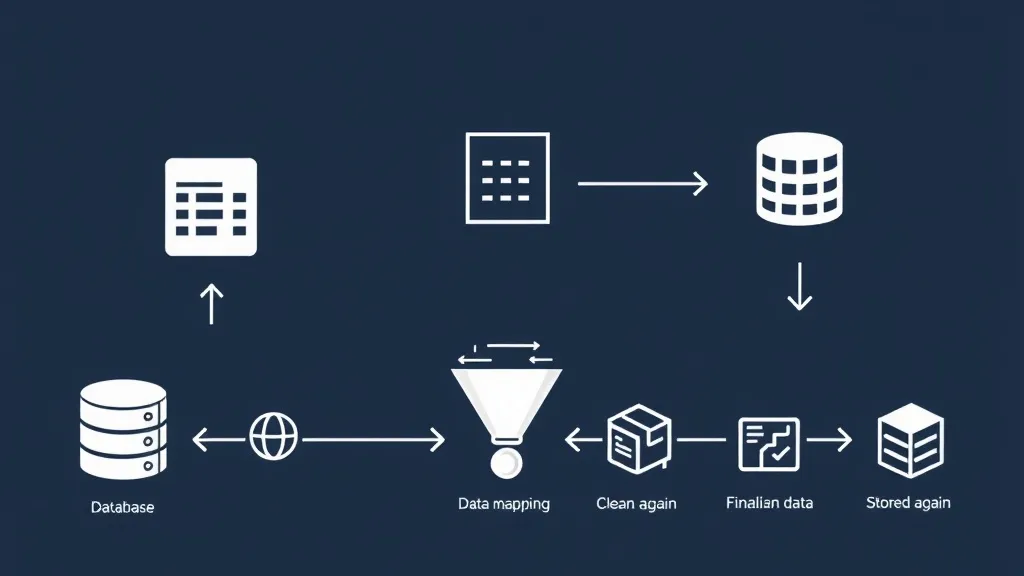
3. Pilot Development & Testing
We developed a reinforcement learning-based AI scheduler that could:
- Optimize job sequences across multiple machines and shifts
- Adapt to dynamic inputs like priority changes or downtime events
- Simulate and recommend better sequences compared to manual plans
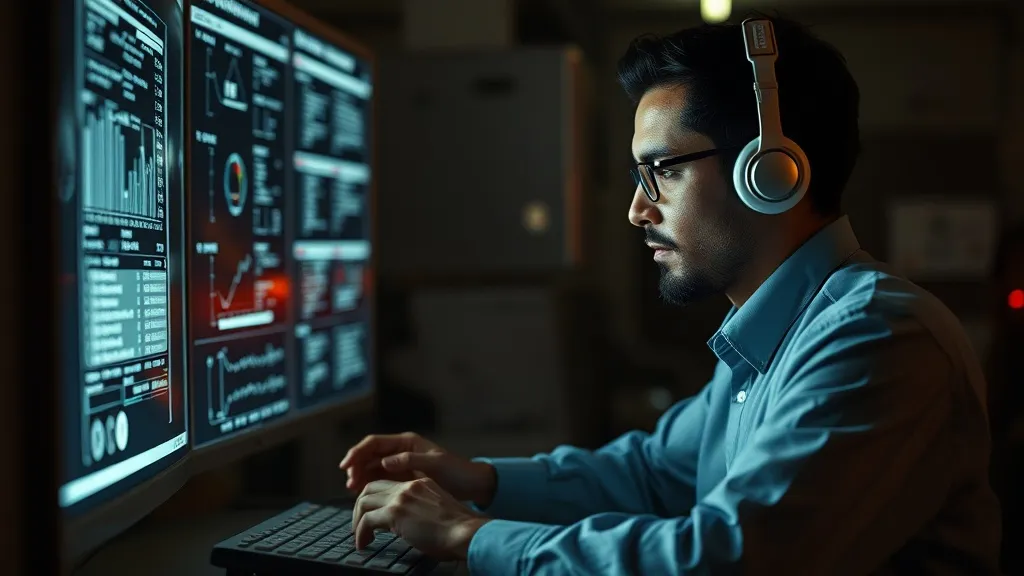
4. On-Floor Validation & Feedback
The client’s scheduling team and line supervisors reviewed AI-generated schedules vs. their own.
- The AI schedules consistently improved machine utilization
- Manual schedule revisions dropped significantly
- Floor teams trusted and adopted the system with minimal pushback
- Feedback loops helped fine-tune priority logic and exceptions
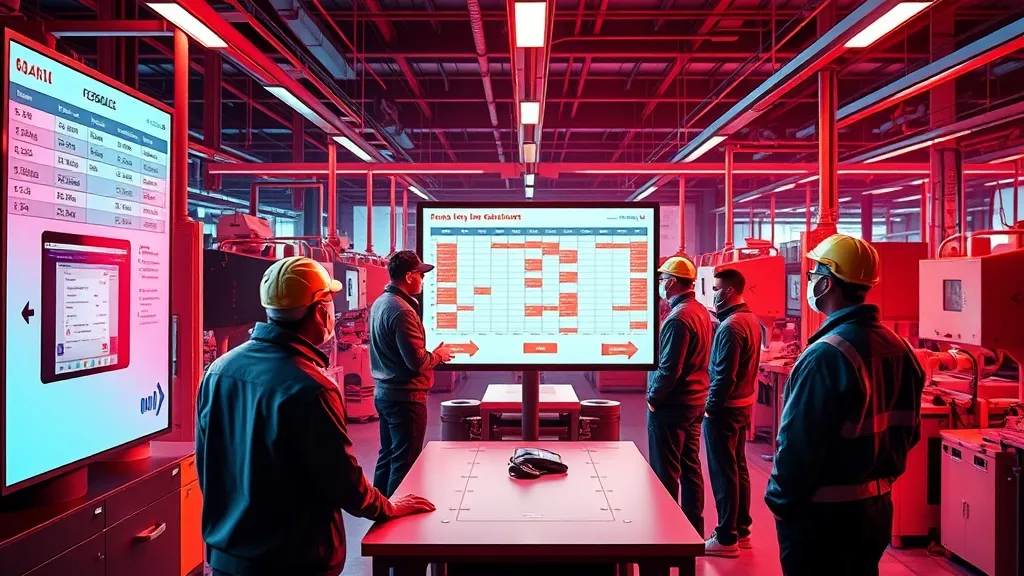
5. Automation & System Integration
With proven pilot results, we are moving towards a full automation:
- Integrating the scheduling engine with the client’s ERP system to pull live data
- Building custom dashboards for supervisors to review and adjust schedules
- Enabling real-time re-optimization based on floor activity and disruptions
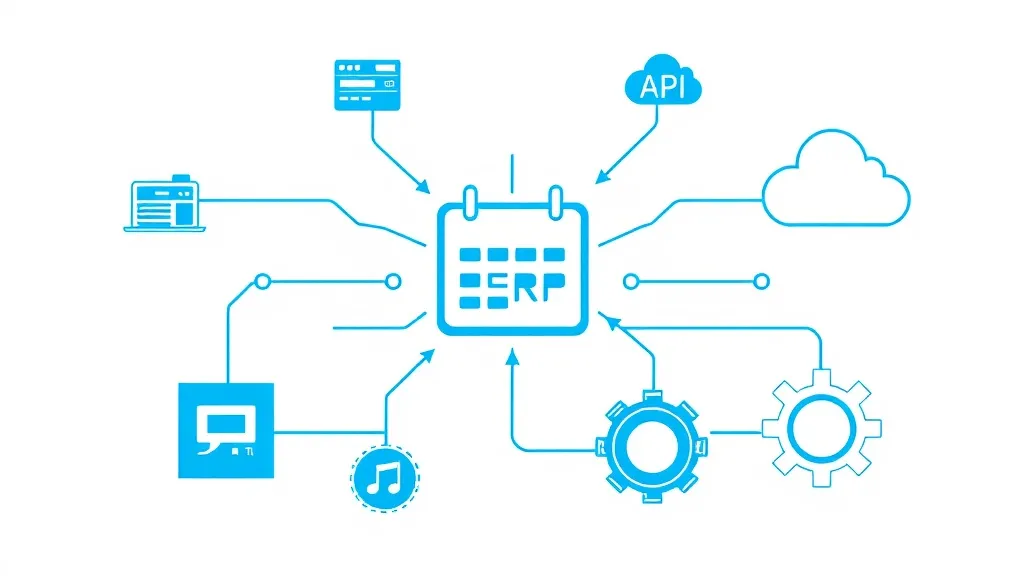
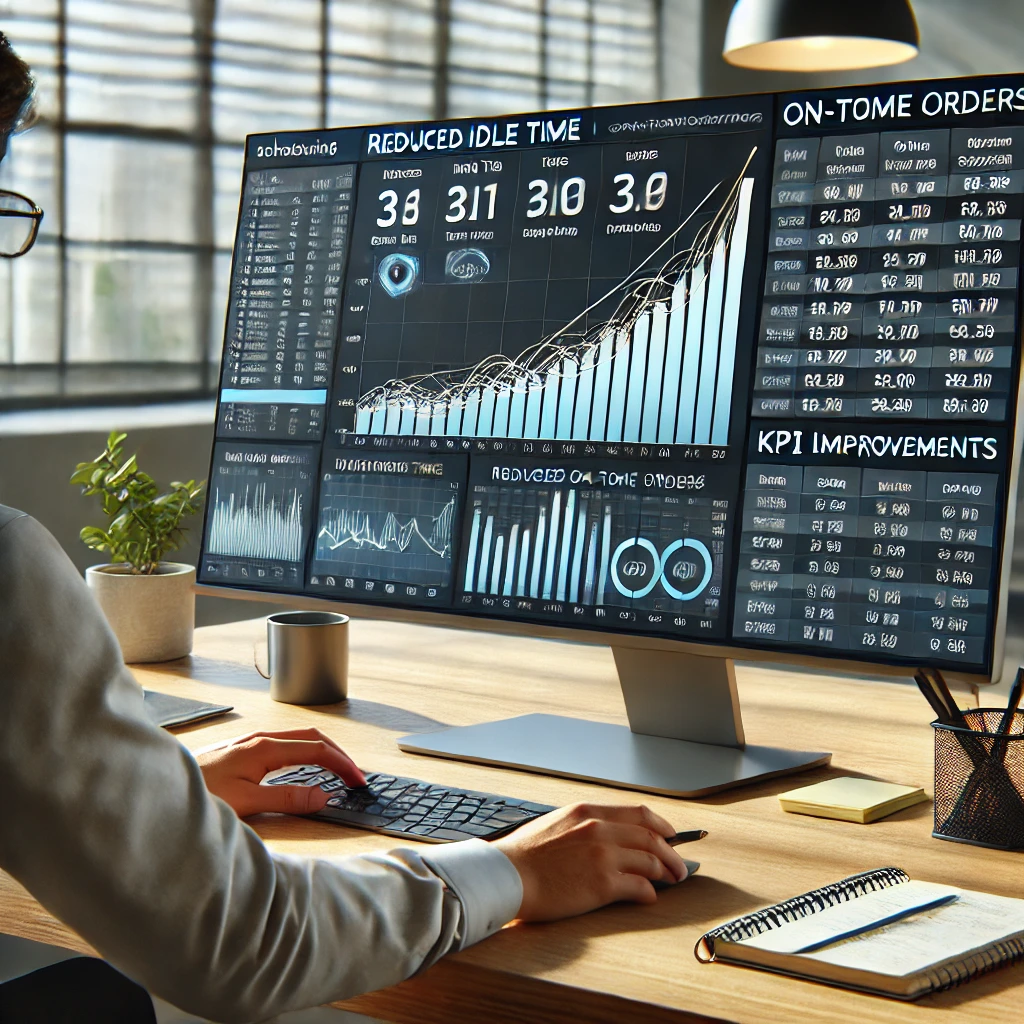
From Firefighting to Flow — Smarter Scheduling at Scale
The AI scheduler transformed how the client runs production — shifting from reactive planning to proactive execution, without changing their ERP or team structure.
- 18% reduction in machine idle time
- 25% improvement in on-time production orders
- 60% reduction in manual scheduling time
- Supervisors now focus on exceptions, not juggling job lists